Opinion | Predictive policing models encode problematic assumptions

[ad_1]
Mathematical models appear like scientific analysis and are often readily accepted as fact. Yet, all models are mere abstractions — they represent processes in simplified ways by taking certain inputs and predicting responses in different situations.
Several assumptions are made in the construction of models and especially when used in the criminal justice system, allowing them to go untested can result in many unintended harmful consequences. While mathematical and machine learning models can appear unbiased, in reality, they encode prejudices against historically marginalized groups.
One such model is PredPol, a software tool that predicts the areas and times crimes are most likely to occur to increase effectiveness and efficiency in policing. Based on historical crime data including crime type, location, date and time, algorithms pinpoint locations where patrolling should be increased.
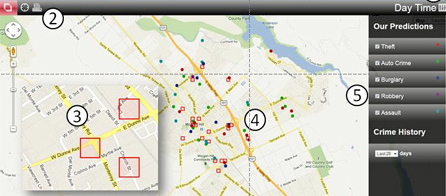
According to the firm itself, PredPol is being used to “help protect one out of every 33 people in the United States.” Given how ubiquitous this crime prediction software is, it is important to scrutinize its methods and test the assumptions it makes about policing and crime prevention.
PredPol targets geography and not individuals. Factors like race and ethnicity are explicitly excluded from consideration. Crime data is also anonymized such that any information that could potentially identify individuals is neither collected nor used in prediction. On the surface, it appears as though there is nothing in PredPol’s calculations that make it discriminatory.
However, within cities where economic and racial segregation are persistent, geography can be an effective stand-in for class and race. In the 1930s, the Home Owners’ Loan Corporation created Residential Security Maps of major American cities based on the mortgage lending risk. This practice of redlining reinforced the segregated structure of cities in the United States.
According to a report by the advocacy group National Community Reinvestment Coalition, 74% of the neighborhoods the HOLC marked as high-risk or “hazardous” are now low-to-moderate income and nearly 64% are minority neighborhoods. Even with directly identifying information removed from consideration, characteristics like class and race are inextricably linked to geography. While likely unintentional, these factors still become deeply embedded into models anyway.
PredPol also makes problematic assumptions about the kinds of crimes that should be targeted and how serious crimes should be prevented. Training documents from PredPol itself indicate that its approach is aligned with the discredited “broken windows” theory. Under this policing paradigm, misdemeanor crimes are viewed as a gateway to more serious ones.
The theory suggests that maintaining order and preventing crime coincide with one another. In the training document obtained via a Freedom of Information Act request, PredPol states that their approach is “oriented towards reducing misdemeanor crime” which in turn “may also reduce felony crime.”
However, this line of reasoning that the software is predicated on has been called into question by several studies. Research published in the Annual Review of Criminology found that there was “no consistent evidence that disorder induces higher levels of aggression or makes residents feel more negative toward the neighborhood.”
Such findings come in direct conflict with the conventional wisdom suggested by the theory. If a goal of PredPol is to stop felony crimes, the inclusion of large swaths of relatively minor or harmless crimes analogous to disorder is not an effective predictor.
The use of nuisance data in the form of misdemeanor crimes is also dangerous in its effectiveness at creating feedback loops. The use of past data to determine where to concentrate police presence is also flawed for this reason.
Feedback loops are essentially self-fulfilling prophecies. They take existing assumptions, apply them, then use those applications to justify the perpetuation of the original assumption. In the case of PredPol, one of the original suppositions is that misdemeanors are a good predictor of future crimes and that areas should be monitored more based on this fact.
Areas with historically high rates of minor offenses are disproportionately poor neighborhoods of color. Since these neighborhoods are already classified as hot spots for crime, police end up monitoring them more frequently.
Serious crimes like murder are likely to be reported no matter where patrols are located. However, relatively minor crimes, like underage drinking, trespassing, vandalism and drug possession, often go unreported.
In hot spots with increased police presence, misdemeanors that otherwise could have gone unnoticed get recorded, adding even more crime data to those areas. This influx of data justifies further policing of that area, and the vicious cycle continues.
Feedback loops created by models like PredPol formalize existing discriminatory patterns, many times without detection. PredPol has a large sphere of influence and has made a tangible impact on policing in areas around the country, all while operating with minimal transparency.
While it seems like a straightforward solution, with some digging, it is apparent that there are many problematic assumptions that it relies upon. This is not to say that a data-driven approach within the criminal justice system is a bad thing. The introduction of data has the potential to create more consistent and fair systems.
But, it is still important to remember that data comes from human experiences. Disregarding such a fact can be dangerous. Big data has a dark side — if models like PredPol are not fully scrutinized, immeasurable harm can be done.
Rayna is a sophomore in LAS.
[ad_2]
Source link